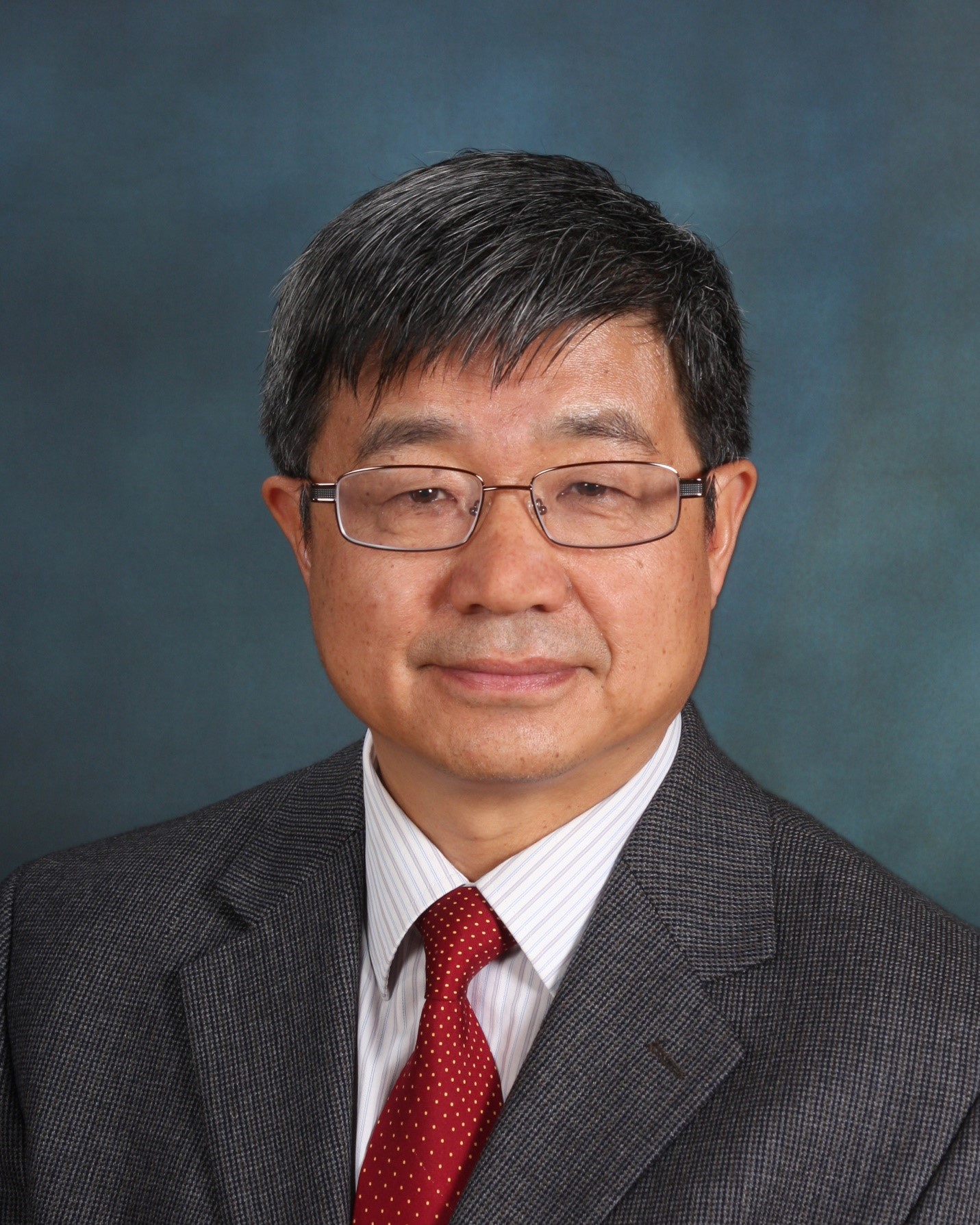
Short Bio: Qi-jun Zhang Fellow of IEEE Fellow of Canadian Academy of Engineering Chancellor’s Professor, Carleton University, Ottawa, Canada www.doe.carleton.ca/~qjz
Qijun Zhang received the B.Eng. degree from the Nanjing University of Science and Technology, Nanjing, China in 1982, and the Ph.D. Degree in Electrical Engineering from McMaster University, Hamilton, Canada, in 1987. He was a research engineer with Optimization Systems Associates Inc., Dundas, Ontario, Canada during 1988-1990 developing advanced optimization software for microwave modeling and design. He joined the Department of Electronics, Carleton University, Ottawa, Canada in 1990 where he is presently a Chancellor’s Professor. He has also served as the Chair of the Department of Electronics, Carleton University 2009-2011. His research interests are modeling, optimization and machine learning for high-speed/high-frequency electronic design, and has over 300 publications in the area. He is an author of the book Neural Networks for RF and Microwave Design (Boston: Artech House, 2000), a coeditor of Modeling and Simulation of High-Speed VLSI Interconnects (Boston: Kluwer, 1994), and a coeditor of Simulation-Driven Design Optimization and Modeling for Microwave Engineering (London, UK: Imperial College Press, 2013). Dr. Zhang is a Fellow of the IEEE, and a Fellow of the Canadian Academy of Engineering. He is an Associate Editor of the IEEE Transactions on Microwave Theory and Techniques, and a Topic Editor for the IEEE Journal of Microwaves. He is the Chair of the Technical Committee on Design Automation (MTT-2) of the IEEE Microwave Theory and Techniques (MTT) Society, and Founding Chair of the Executive Committee of the IEEE MTT Society’s annual international conference on Numerical Electromagnetic and Multiphysics Modeling and Optimization (NEMO). He is the Guest Editor of the upcoming Special Issue on AI and Machine Learning for Microwaves for the IEEE MTT Transactions, scheduled for publication in 2022.
Speech Title (on-line): Machine Learning for Microwave Modeling and Design
Abstract: This talk describes machine learning techniques for microwave modeling and design. Artificial neural networks are trained to learn the behavior of microwave passive and active devices and circuits. The trained neural networks become fast and compact behavioral models for high-level circuits and systems design. Knowledge-based techniques combining microwave knowledge with neural networks allow us to use machine learning to modify existing models into new ones. Artificial neural networks are also trained to learn inverse problems in microwave design providing direct solutions to inverse microwave problems where direct formula is not available. Examples of artificial neural networks for parameterized modeling and optimization of electromagnetic structures, and for modeling of active microwave devices and circuits will be provided to demonstrate machine learning for microwave modeling and design.
|